The question of how we engage with technology has persisted throughout art history, from concerns about photography displacing painting to debates around mechanical reproduction. Today, I find myself navigating similar philosophical terrain through my work with generative technologies. I use AI across different areas of my practice, neither rejecting technology outright nor embracing it uncritically. On the pragmatic side, I generate bureaucratic text for grant applications and tedious letters—documents intended for no one in particular. Perhaps two lawyers will read them when judging my application, but they require a description nonetheless. For this kind of text, I don’t hesitate to use AI: it’s quick, and hardly anyone reads it carefully. But if I needed to write something important—something involving feelings, nuance, and precision—I would never use an algorithm. That I would write myself. When it comes to creating images, AI connects deeply to artistic traditions I feel aligned with. Coming from a Fluxus tradition, through John Cage, surrealism, and automatic drawing, I see clear parallels between historical practices and using systems as collaborative partners. The Fluxus movement’s collapse of boundaries between art and life, and Cage’s embrace of chance, prefigured today’s moment, where algorithms become co-creators. Just as I might shape a sculpture while letting plaster and materials exercise their agency, I can engage an algorithm as a partner in creation. This approach challenges the romantic idea of the solitary genius creating from nothing. If you don’t subscribe to individual genius, but instead see yourself as part of a collective process, these technologies make sense. AI simply makes visible what has always been true: creation is dialogue, not monologue. Of course, my relationship with AI is not without tension. When these tools first emerged, my initial reaction was irritation—yet another big-tech invention, more advertising imagery made by people I had little respect for. But at the same time, they aligned with my interest in how we create narratives and understand language. They also offered practical benefits. Economically, I couldn’t afford to hire a team of photographers for a specific project, but with AI, I could create the images myself. Technology democratizes production while simultaneously serving capital and power. This brings an economic question into focus: who controls the means of production—the artist or the corporation? We are living in an era where manipulation and recontextualization define creativity. At the same time, these algorithms are deeply problematic. They are biased, favoring American aesthetics, and some companies behind them are already selling to the defense industry. But if we see AI not as a creator but as a filter of human material, then the outputs remain human—remixes where content, style, and technique become fluid variables. I have always found the art world’s focus on individual genius ill-suited to my practice. The troubling questions AI raises about authorship and ownership feel like necessary disruptions. They force us to confront the mythology of originality that has haunted Western art since Romanticism. The question “What is an author?” becomes even more pressing with AI. Is the work mine? In one sense, yes—I have shaped much of it. But it is also collective. This doubleness seems the most honest way to describe the process. Even painters with twenty assistants or artists clearly working within traditions are participating in collective creation. Art has always been collective, despite the myth of the lone genius. The art world’s authority has long rested on authenticating originality and assigning value based on scarcity. AI fundamentally challenges both. When a machine can generate endless variations based on the entire history of art, what constitutes the “original”? When digital abundance replaces material scarcity, how do we assign value? These aren’t new questions—Duchamp and the appropriation artists raised them too—but AI intensifies them for contemporary art institutions. I would rather be transparent about using AI than pretend otherwise. There’s a freedom in honesty. If I tried to conceal my use of algorithms, I’d panic if asked about it. Instead, I can open the door and say, “Yes, I used this algorithm, and I wrote this prompt. What would you write?” That openness is liberating. Economically, the implications for artists are significant, especially for those relying on traditional mediums like painting. AI disrupts established economic models and raises familiar historical questions: who benefits from technological change? Who is displaced? If AI can replicate an artist’s style with ten examples, it’s not only an aesthetic issue but an economic one. Capitalism continually revolutionizes production while destroying existing structures. If universal basic income were available, this might not matter—but within a system that relies on scarcity, it becomes a real concern. The art world is wondering: if anyone can generate images, what happens to the traditional hierarchies? Democratically, that’s exciting—but it also threatens those who previously held privilege. Technology could democratize production, but the economic structures reinforcing inequality remain intact. Today, a handful of artists dominate Denmark’s art market while many others struggle. If redistribution meant some artists earned a little less while others earned a little more, I would welcome that. But in practice, AI is hollowing out the middle—artists who once sold enough to survive are increasingly unable to sell anything. This situation raises fundamental questions about how we value artistic labor in a post-scarcity image economy. When the means of production are democratized but economic structures still rely on artificial scarcity, a profound contradiction emerges—one that demands social, not just technological, solutions. I don’t see AI image-making as a radical break but part of a longer evolution. Media technologies have always developed incrementally. When I take a photo on my phone, algorithms already apply noise reduction and color adjustments before I even see it. Almost no image today is untouched by computation. The boundary between “real” and “artificial” images is not an ontological one but a political one—a way of preserving certain hierarchies of knowledge and authority. AI brings old debates about authorship and authenticity into sharp relief. The surrealists' exquisite corpse games are echoed in today’s algorithms: assembling heads from one source, hair from another. It’s collective creation all over again. AI does not break with the past so much as it makes visible processes that were always there: creation as recombination rather than invention ex nihilo. Originality has always been a myth; AI simply exposes it. Rather than flee technology or embrace it naively, I prefer to stay inside the system and ask: what kind of image-world do we want to live in? This means resisting algorithmic homogenization, creating counter-images that reflect local realities instead of generic international standards. Global digital culture tends to “smooth out” difference, turning everything into variations on familiar themes. AI generation, with its American visual bias, is part of this smoothing. Insisting on local specificity becomes a political act. I find the moment of “suspension of disbelief” in AI images fascinating—the moment when you almost believe the image is real, before noticing the extra finger or a glitch. This spectral quality—simultaneously believable and unbelievable—reflects how I see photography itself. Photography has always had a compromised relationship with truth. AI just makes the construction more visible. I aim not to mystify my process but to share it openly. When you look at one of my images, I want you to think, “I could make that too.” I’ll gladly tell you what algorithm I used, what text I wrote. There’s strength in collective knowledge. This openness also challenges the art market’s logic of scarcity. By open-sourcing my methods, I try to resist the commodification of creative knowledge and maintain creative autonomy. I run AI models locally on old hardware, powered by wind energy—a practice I call “permacomputing.” It allows me to bypass corporate filters and to work more sustainably. Local computing imposes limitations, but it also restores some human scale to digital creation. There’s embodied knowledge in this too: the heat generated by my computer now warms my workspace. Feeling the physical energy demands of computation reminds me of the infrastructures normally hidden by slick interfaces. Cultural bias remains a persistent problem in AI image generation. Trying to generate Danish scenes often results in stereotypical German or Dutch imagery. Even when typing “Denmark,” the output looks wrong. Ironically, requesting “Solvang”—a Danish-themed town in California—produces better results. These systems reproduce cultural frames embedded in their training data, often invisibly. It’s like explaining reality to a drunk American—they listen, but only halfway. I’m concerned by how ubiquitous AI-generated images have already become—on buses, in ads, everywhere—often unmarked. These polished, culturally-biased images risk shaping our sense of reality itself, replacing lived experience with idealized simulations. This leads to copies without originals—representations that become more “real” than reality. When simulations precede experience, we must question what remains of authenticity. My process involves proposing theoretical concepts and observing how algorithms interpret them—working with available materials to create new meaning. For instance, I once theorized that hip-hop arrived in Denmark via fishing fleets, drawing from my wife’s father’s stories of a Swedish-Danish maritime language. When I asked the algorithm to visualize this, it produced images of people dancing on ships in storms—an unexpected but fitting outcome. This shows how technical systems participate in creation, not just as tools but as agents that shape meaning through their resistances and biases. The final work emerges from a dialogue, neither wholly mine nor the machine’s. The “mistakes” these systems make—like extra fingers—reveal cracks in their logic. They point to aspects of reality that resist idealization. I value these ruptures: they show where systems fail, and where something new might emerge. These glitches reveal something interesting. When algorithms attempt to create perfect digital images but fail, the resulting imperfections often appear more authentic than the polished successes. The additional fingers or distortions show the constructedness of these images, which is actually more representative of reality than idealised perfection. What maintains my interest in this practice is not uncritical acceptance or complete rejection of these technologies, but working in the collaborative space between human and machine creativity. In this area of collaboration, neither human nor machine has complete control; both contribute to the final result, often in surprising ways. The algorithm works according to its parameters and training. We, in turn, interpret its outputs, question its assumptions, and find interest in its limitations. This mutual interaction creates new forms of aesthetic practice that don’t eliminate human creativity but extend it in different directions. As these technologies continue to develop, the important question isn’t whether AI will replace artists—it won’t—but how we structure our relationship with it. Will we give up our agency to corporate platforms driven by profit? Will we permit algorithmic standardisation to reduce cultural differences? Or will we continue to engage with technology on a human scale, preserving diversity, and democratising production and economic value? The project about hip-hop and fishing fleets demonstrates this collaborative process effectively. The images of people dancing on ships in storms weren’t my original idea, nor were they simply the product of the algorithm’s dataset. They emerged from our interaction. Neither of us planned this outcome, yet it effectively conveyed something interesting about cultural mixing, creative adaptation to difficult circumstances, and the unexpected links between different communities. This illustrates clearly what artistic practice involves in our current technological context: adapting to technological changes whilst maintaining our creative identity, finding flexibility within constraints, and developing new approaches as the conditions change.
Reflections on AI and Artistic Production (en)
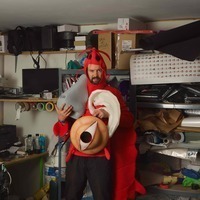
Kristoffer ørum
@Oerum